Introducing Data Lakes for Conversational Data
Companies that leverage their conversational data today will quickly outpace competitors. Introducing Airy Conversational Data Lakes: Enabling your company to store and utilize conversational data.
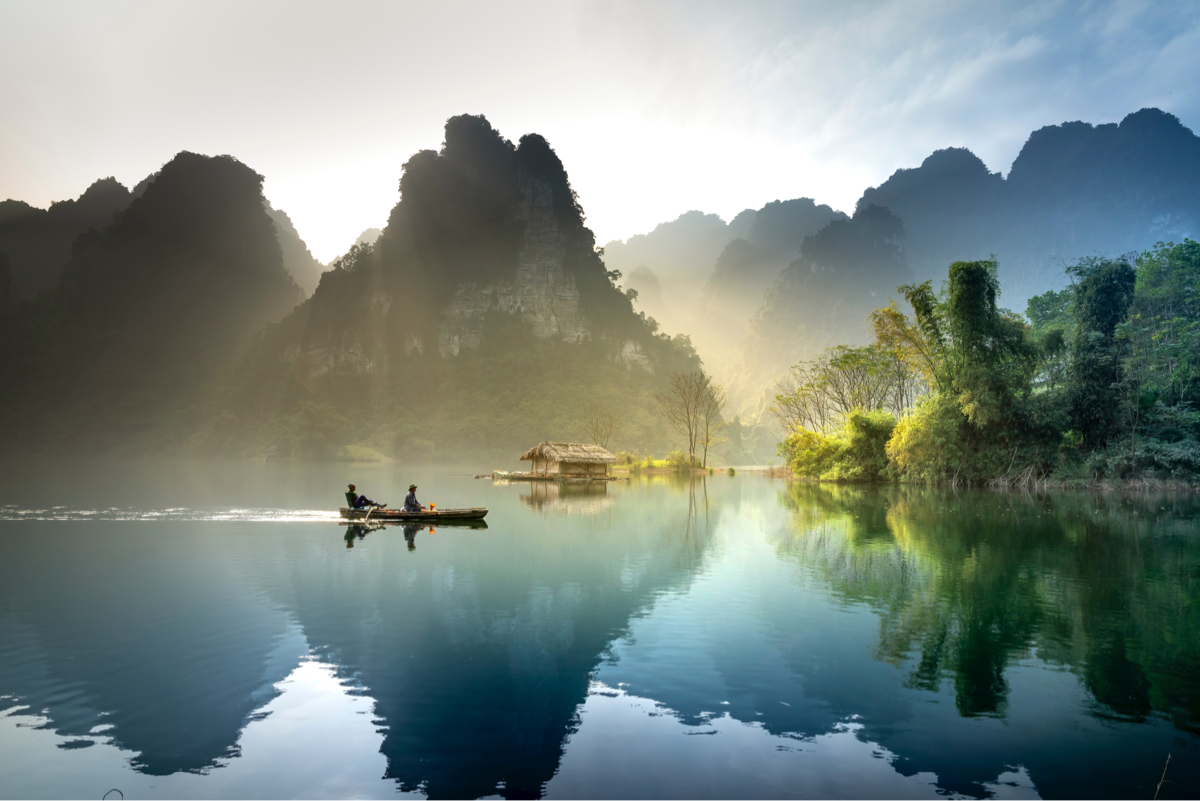
Data Lakes have continued to cement their strong position in today's data driven world. More and more companies are benefiting from separating their needs to store data and their needs to analyze it. The data lake solutions provided by current cloud providers are mature, cost-efficient, incredibly versatile and future-proof.
What are Data Lakes?
Essentially, data lakes are a place to store all of your data for analytical purposes, much like a data warehouse. The easiest way to differentiate the two, is that a data lake does not include the means to do any sort of calculations with it. It is nothing more than a very reliable and infinitely scalable object store. The benefit of that, is that you only pay for the storage you use. Unlike a data warehouse, where you either pay for empty hard disks or idling server resources.
Meanwhile with a data lake, where storage and compute is decoupled, you can leverage the unstructured nature of data lakes and import data in any shape, volume or form.
Furthermore, you can choose to enable crucial features by ticking a few boxes, like encryption and data livecycle policies, for when you want to save even more money on less frequently accessed slices of your data.
But, because storage alone doesn’t provide any insights, data lake providers have worked hard to integrate well with the existing analytics solutions out there, enabling customers to use the tools they are already familiar with, like SQL and Apache Spark.
What makes Conversational Data special?
At Airy, we have been processing conversational datasets for many years and have seen the exponential growth of this new domain for customer-business conversations. With the shift to messaging, more and more customer interactions, from pre-purchase questions to customer service requests, become conversational.
The data itself has a high signal vs noise ratio, since inside of the data you can find answers to all sorts of questions, from findings about bottlenecks in internal company processes to preferences of customers.
Unfortunately, conversational data is currently highly siloed: customer service chats are often exclusively stored inside the customer service database, or pre-purchase related questions stay within the tools that social media teams use to engage with people on social media channels.
Because there are a multitude of different messaging providers that structure their data differently, it is hard to aggregate this data in a way that enables economical storage and still provides the ability to do powerful analytics in a variety of tools.
Conversational AI, spearheaded by teams like Rasa and Dialogflow by Google, aims to automate many conversational use cases. Finely grained real conversational data makes it possible to train models in real time.
We have seen entire customer service departments overhauled, as conversational data has been successfully used to train models in order to automate FAQs, freeing agents to tackle more complicated requests, further improving response times and customer satisfaction.
Airy’s Conversational Data Lakes on AWS
On AWS, conversational data can be stored in Amazons S3, providing for a cheap storage option for massive amounts of data. To optimize for performance when querying data, raw AVRO formatted conversational data is converted into compressed Apache Parquet files.
To eliminate manual schema management, we also provide schema information with our AWS Glue data catalog integration. With this, you can get a clear overview of all conversational data, down to the last column, and query it instantly with AWS Athena.
From here, it's very easy to integrate in all the standard tools that the data science and analytics communities love.
Encrypted by default
For regulatory compliance and data protection it is often required to encrypt data at rest. We handle this with Amazon S3-managed encryption keys which means your data is secured with AES-256 block cyphers before it is written to disk. Arguably even more important is to also encrypt your data in flight because it then leaves your infrastructure and is sent to S3. For this case we support SSL/TLS encryption to prevent anybody from eavesdropping on your traffic.
Future-proof for advancements in conversational experiences
Conversational providers such as WhatsApp and Facebook Messenger are constantly innovating, varying their feature sets and offering different use cases. To stay on the leading edge our engineers have developed a data model achieving symbiosis of structured and semi structured data.
Our highly scalable ingestion platform, based on Kafka, streams the data into our system where the conversational data from all providers is resolved into uniform schemas of channels and messages.
We also support unstructured metadata throughout our system for when additional information is required. An example of this metadata would be internal open-done states, clearly showing in a UI if a conversation needs a human response.
Integrating into your existing Data Lakes and Warehouses
The real value of your conversational data becomes apparent when you merge it in real time with your existing data, creating a more complete model of your customers.
If you have a lot of existing data, your Airy Data Lake integrates seamlessly with analytics tools like Apache Spark for powerful analytics at any scale.
The Power of Conversational Data Lakes
Understanding your conversational data better is essential for providing your customers with the best possible service. From identifying patterns in your customer support cases and analysing customer preferences to training your Conversational AI model with Rasa or Dialogflow, it all becomes easily accessible with a data lake.
In a recent case with one of our large retail customers, their Airy Conversational Data Lake has enabled them to do a deep dive on their Google Business Messaging data to improve their customer satisfaction rating.
By combining the results of the Customer Satisfaction Score (CSAT) with the source data on a per conversation basis, it is possible to pinpoint exactly which kind of conversations are leading to lower scores. With these insights, solutions can be precisely designed and implemented, leading to greatly improved customer satisfaction and reduced customer churn, all with minimal cost and effort from the company’s side.
Visualization is also just a query away: This simple breakdown shows CSAT by the day of the week. It’s immediately clear that the majority of negative scores are reported for conversations beginning towards the end of the week. The customer service department of this particular company is comparatively understaffed over the weekend, leading to increased customer waiting times for a response. Percentage wise, dissatisfaction is at its highest over the weekend.
Using a simple visualisation like this, one can glean that a few more service agents on duty on Saturdays could improve CSAT dramatically.
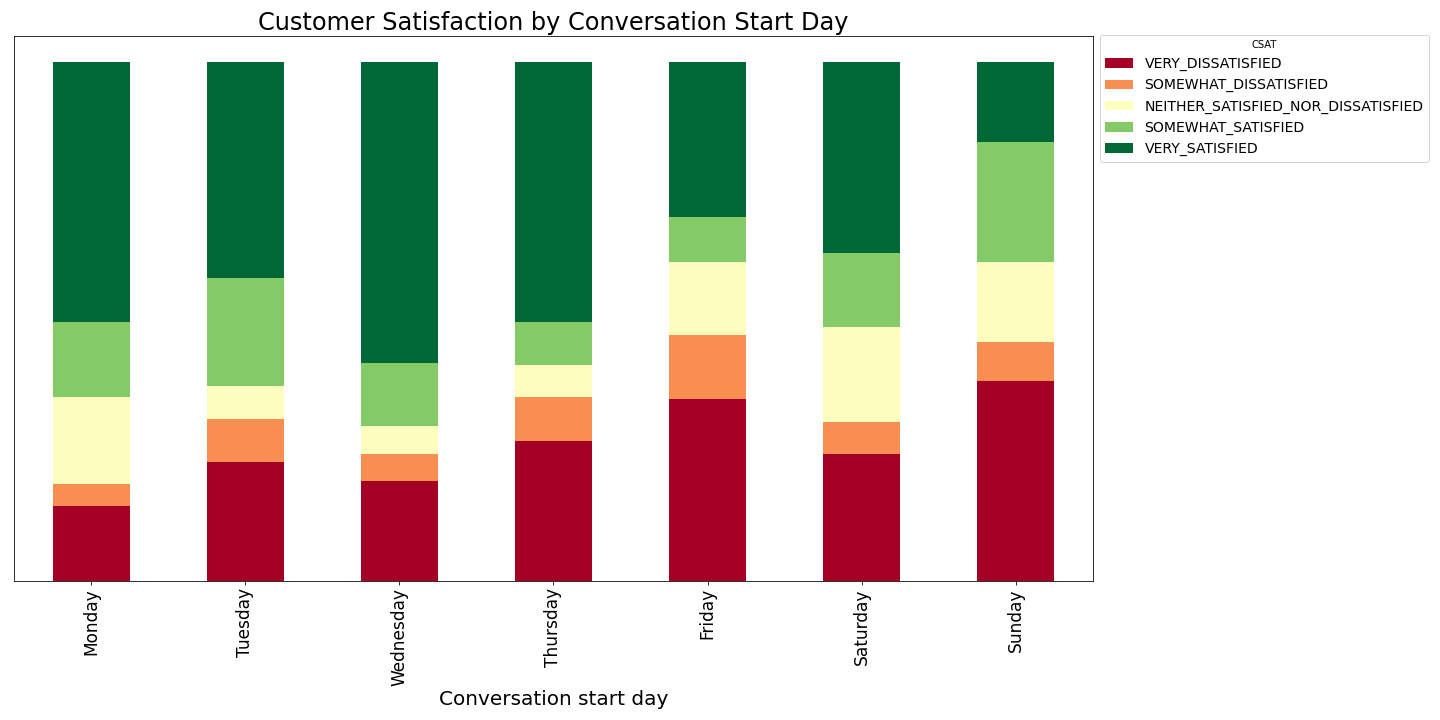
Key Benefits of Conversational Data Lakes
Setting up a Conversational Data Lake now (or integrating conversational data in your existing data lake) sets your company and data teams up for a future where most customer and business interactions are conversational and automated by Conversational AI. Having your own conversational data lake leads to:
- Much lower costs for data storage (compared to most data warehousing solutions)
- Immediate insights into customers' preferences and behavior
- Reduced engineering times for data scientists
- Readiness for the exponential growth of conversational data & your own conversational AI models
Get started today!
Airy’s Conversational Data Lakes is available to all Enterprise Customers. Get started today with our Quickstart Guide.